( The diagram above ties all the individual essays together, it's helpful to keep it in mind as you read through.
Complementing this diagram and a necessary reading before proceeding with any essay in the publication, including this one, is the Reader's Guide. It is aimed at helping readers understand some of the concepts developed throughout the entire publication and avoiding repetition between the individual essays. Please look at it now, if you haven’t already; you can also review the guide at any later time through the top menu on the publication's home page.
If the Reader's Guide functions as a Prologue, the post Personal AI Assistant (PAIA) functions as an Epilogue; in that post, we begin to construct a formal approach to democratic governance based on the PAIA. We may even go out on a limb and attempt to pair that democratic governance with a more benign form of capitalistic structure of the economy, also via the PAIA.
There are two appendices to the publication, which the reader may consult as needed: a List of Changes made to the publication, in reverse chronological order, and a List of Resources, containing links to organizations, books, articles, and especially videos relevant to this publication.
The publication is “under construction”, it will go through many revisions until it reaches its final form, if it ever does. Your comments are the most valuable measure of what needs to change. )
What should AI regulation aim for, in general terms? It should aim to uphold what we called the reconciled values in the Democratic Principles versus Human Values article, i.e. a non-contradictory set of democratic principles and human values. We saw in that article that democratic principles and human values can be contradictory and we postulated that this set of reconciled values exists; see Axiom 2 at the bottom of every post in this publication. So when we use the terms values we mean this reconciled set. Secondly, recall from Appendix 1: Reader’s Guide that human values take precedence over democratic principles.
Let’s set AI regulation within the larger context of this publication. We have postulated many times, e.g. in the formal system at the bottom of every essay, but more pointedly in Man's Search for Relevance, that a new kind of citizen will emerge in the near future (some say within the next five years), a citizen endowed with a personal AI assistant (PAIA) running on personal devices, or in cyberspace, or more probably in both. Whether this assistant may have an actual physical aspect (be a robot) is not relevant here.
Let’s also keep in mind that your PAIA will participate in that AI alignment of human values and refine and strengthen that alignment with your own values, as we make the point in the AI Language Models as Golems article.
What is most relevant to us in this publication is the interaction between YOU, the PAIA, and a democratic government. The main idea is that YOU are (or should be) smack center of that interaction, and that the other two are at your service. But to avail yourself of those services, you have to participate.
That’s why I put the quote by Ann Richards, the legendary former governor of Texas (a woman and a Democrat governor of Texas, yes, you read that correctly!), in the short description of the publication, the first thing a new reader sees when opening the publication.
Let’s put those three elements, YOU, your PAIA, and the government, into a triangle and keep that triangle in mind as we speak about AI regulatory action by the government. We will try to understand the relationships between the three components of the triangle, and their role in setting and following AI regulations.
Setting the Tone: Regulating AI is a Serious Matter
There is probably no better introduction to the seriousness of this matter than the following interview with Geoffrey Hinton.
Upholding Values
Regulating AI so that it does indeed uphold the reconciled set of values obviously cannot be a one-time action, but an ongoing process. We should look at regulation as part of a larger goal, towards a future where AI, democracy, and human values, not only coexist but synergistically enhance one another, as reflected in the top circling diagram of this (and every) post.
Strong AI regulations are not yet in place and therefore, calls have been made to pause or slow down AI development until they are. Some even more alarmist warnings have been issued about AI potentially leading to human extinction. I am not a signatory to any of these calls, believing that these scenarios are overestimating the current stage of AI development. But the reader should see this side of the argument (which by the way is in agreement with Geoffrey Hinton’s interview you saw above):
Upholding Values during Development versus Governmental Regulation
To harness the benefits of AI systems while mitigating the types of risks you saw in the clip above, a responsible approach to their development and deployment is obviously required. This responsible approach includes ensuring transparency in AI algorithms and actively working to reduce biases in AI models.
In the case of the LLMs, we also introduced the RLHF method and emphasized the central role of this method in aligning the generated responses with human values. Involving a diverse range of stakeholders in the development process can also help in aligning these models with a broader range of human values.
But apart from this alignment done in the industry during development, separately establishing robust legal frameworks (what we call regulation) to verify AI-generated content is also necessary and it must obviously be done by the government, not by the developers of AI. Let’s keep in mind this separation of concerns between industry and the government as we move along.
Educating and Empowering the Public
What about the public? In this entire publication we are concerned with the interaction between AI, democracy and human values. So clearly, not just developers and the government need to be involved in the AI process. Equipping the public with the knowledge to discern AI-generated content and understand its implications is equally important. Educational initiatives that focus on digital literacy and critical thinking can empower the public to use AI systems more effectively, make informed decisions, and participate actively in shaping AI's role in society. This is important in ensuring that the public remains the ultimate arbiter in democratic societies, and not swayed unduly by AI-generated content.
Finally, cultivating a collaborative approach between AI developers, policymakers, and the public is vital. It is only through such a collaborative approach that a harmonious coexistence of AI and human society can emerge and the true potential of AI can be realized.
The Two Best Known Regulatory Acts
The two best-known regulatory acts are the European Union (EU)'s AI Act and the United States' upcoming AI Bill of Rights. We look now at the balance of concerns and approaches between the two because they are quite different.
European Union AI Act:
Risk-Based Approach: The EU AI Act categorizes AI systems based on the risk they pose, with different levels of regulation for each category. AI systems considered a threat to people will be banned, while high-risk systems will face stricter regulations.
Transparency and Accountability: Generative AI, like ChatGPT, must comply with transparency requirements such as disclosing AI-generated content and publishing summaries of copyrighted data used for training. High-impact general-purpose AI models will undergo thorough evaluations.
Ban on Certain AI Uses: The Act prohibits specific AI applications deemed to pose unacceptable risks, such as social scoring, emotion recognition in workplaces, and biometric categorization systems that infer sensitive data.
Global Impact: The Act will apply to providers and users of AI systems in the EU, irrespective of their location. This means AI developers outside the EU must comply if their systems are used within the EU.
Fines and Compliance: Non-compliance can result in fines up to €35 million or 7% of global annual turnover, depending on the violation.
So one can see that the EU AI Act represents a significant move towards regulating AI at a more granular level, ensuring its safety, transparency, and ethical use. Here is how it has been presented inside the EU:
United States AI Bill of Rights:
Focus on Rights and Freedoms: While specific details of the upcoming AI Bill of Rights in the US are not as fleshed out as the EU AI Act, the Bill is expected to focus on protecting citizens' rights and freedoms in the face of AI advancements.
Comparison with EU Approach: The US approach is anticipated to differ from the EU's, potentially focusing more on guiding principles rather than binding regulations.
So to summarize the differences between these two acts, while the EU AI Act sets a comprehensive legal framework, the US AI Bill of Rights will offer a milder and more principle-based approach, focusing on safeguarding the democratic principles and human rights, hopefully without stifling innovation.
We should also mention that some in Europe worry that the difference between the milder AI Bill of Rights and the stronger EU Act will lead to a continuation of the current advantages the US high tech has over its EU counterparts. Both sides are aware of the negative effects over-regulating AI would have and we will most likely see effective cooperation in the end, after some initial posturing.
AI Regulation in China
Although our focus in this publication is on democratic governance, we should say a few words about AI regulatory status in China, because China is making remarkable progress in AI, and AI systems have the potential to cross national boundaries. China has made advancement in AI a national priority and consequently has been very proactive in setting its own AI regulations. However, regulatory legislation in China is more political in nature. The set of reconciled values (between democratic principles and human values), which has been at the core of our discussion in this publication, has to be subsumed in China to the party values established by the CCP (Chinese Communist Party). Since a discussion of such party values is not within the scope of this publication, we refer the reader to the article Beijing Pushes for AI Regulation published in the Foreign Policy journal.
A Concrete Regulation Picture to Keep in Mind
We are going to use again the LMMs as concrete examples of AI models. They are in fact what many people are thinking of when they think of AI. In the article Language Models as Golems, we saw that building such an AI model is a sophisticated process, consisting of seven distinct steps. We saw in Step 6 of that process that a model’s performance is rigorously evaluated using a variety of benchmarks and tests, including both automated metrics and human evaluations. And we condensed that Step 6 in the following picture:
All the activity present in this picture and in all the other steps of building a model are done by the high tech AI builders: OpenAI, Anthropic, Google, Microsoft, etc. We are now going to adapt this picture to governmental regulatory work. Instead of high tech controlling the evaluation process (as in Step 6), it will now be the government controlling the regulatory process. Keep in mind that not much of this work is being done currently, so we are anticipating here. This anticipated regulatory process is shown below:
The regulatory prompts are designed by the government with the express purpose of testing compliance with the law. The evaluation of the responses given by the model is done either by people working for the government or by automated processes controlled by the government.
LLMs Trained with Public Input
A different approach to RLHF has occurred more recently and it is of great interest to us. It has started with work done by the Collective Intelligence Project (CIP). They used Anthropic's Constitutional AI work as a starting point for their research on the use of public input to direct the behavior of an LLM. The work is explained in the article Collective Constitutional AI: Aligning a Language Model with Public Input.
The model that CIP produced was trained with written input gathered from 1,000 American citizens. The model worked as well as the baseline model in understanding natural language and showed less bias across various disciplines. It is a promising blueprint for people-powered governance of AI and there is hope that other democracies will use this blueprint to create their own versions of models that meet their specific national needs.
Future AI Regulations Will Need Precision and Clarity
Regulating AI is a complex challenge, given AI's unique capabilities. We used the LLMs as an example to appreciate this complex challenge. You can see in the above picture that the people in charge of that regulatory process would have to combine knowledge of the law (the US Constitution and the coming AI Bill of Rights, current congressional regulatory legislation, and any other applicable AI laws) with a technical knowledge of AI.
The compromises made to ensure that the reconciled set of democratic principles and human values is adequately represented makes this regulatory challenge into an even bigger one. We go a bit on a limb now and discuss something that is far from being the accepted (or even discussed) norm. We will argue that because of this complexity, future AI regulations will eventually have to be expressed in a formal, mathematical language, enabling precise verification of compliance. Too much is at risk with AI to allow us to proceed in less rigorous ways.
This formal work would require collaboration between AI research experts and legislative bodies. Formal processes are already in practice for other critical software systems, where there could be large potential financial loss or potential loss of life. So this should certainly include most LLMs, given the possibly catastrophic implications of unregulated models (including as some people believe, the extreme scenario of human extinction).
Aiming for Precision and Clarity through Formal Proofs
The concept of encoding regulations into a formal language and utilizing proof assistants to validate AI systems against these specifications has many difficult hurdles to pass. Our language below will get a bit technical, but hopefully still understandable. This formal approach, known theoretically as “programs as proofs”, would represent a paradigm shift from the normative methods currently in use. In software engineering practice it is known as “formal software development” and if you are interested, I describe it in the presentation below; the link to it is in the caption. It is quite technical.
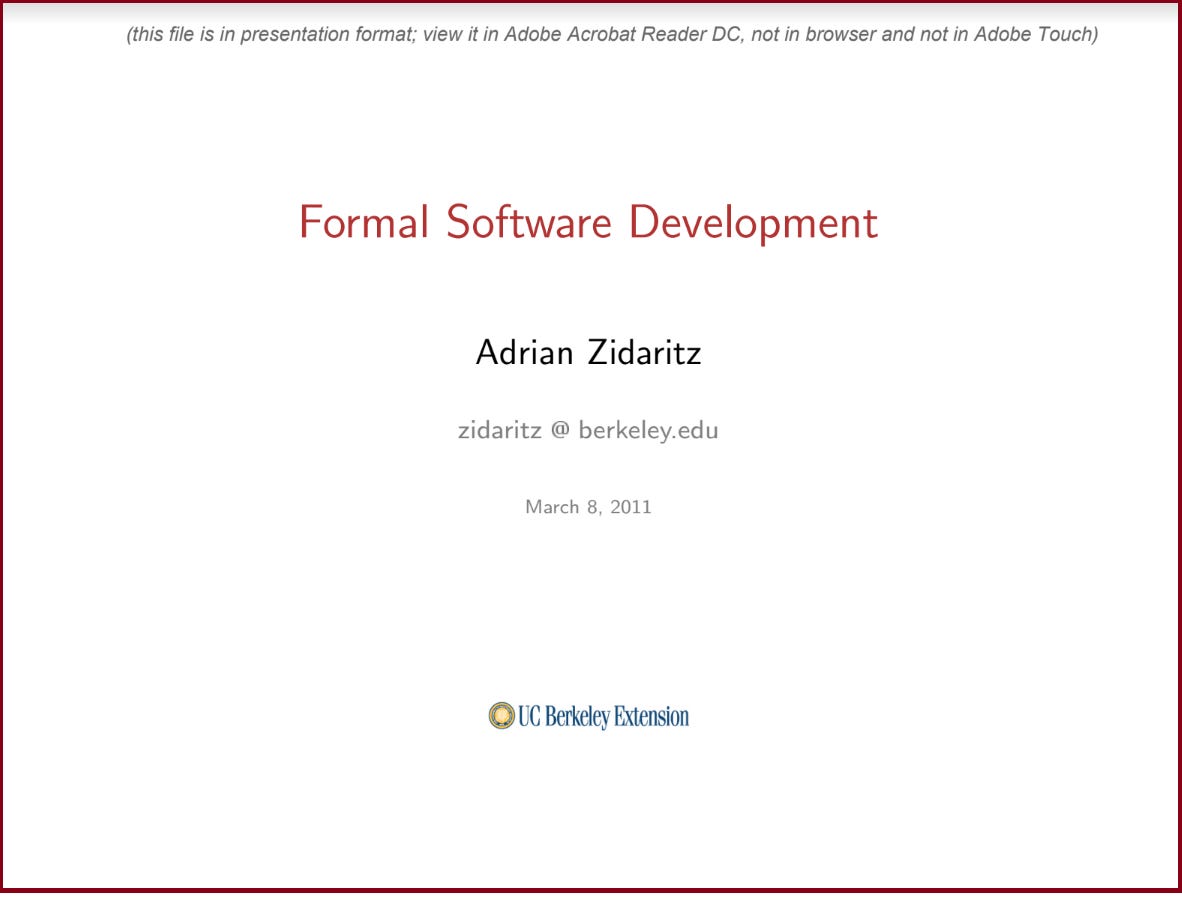
Obviously this method promises enhanced precision, reducing ambiguities inherent in human language interpretations. Here are the most obvious difficulties with this method, as applied to AI. First of all, it would require the development of formal theories that would explain how learning takes place in neural networks.
We do not understand neural networks, which most of the successful AI is based on, i.e. we do not have a mathematical theory of how they learn from data. There is a lot of promising activity in this area, but we are not there yet. I explain all these theoretical difficulties in the article Foundational Questions on the Artificial Intelligence, Dreams and Fears of a Blue Dot website.
Assuming that such a theory becomes available, verifying AI systems would require a high level of engineering expertise, and therefore be very expensive to do. No one will envisage that an entire AI system will have to be developed formally, that just would not be good engineering. Good engineering requires compromises, so what we envisage is that the core of an AI system should eventually be formally developed, while other components can be verified using traditional methods of software quality assurance.
This partitioning out of a core, where any bug is unacceptable, and could potentially have catastrophic consequences, happens in many other areas of software development. One of the best-known examples is that of a formal operating system (OS) microkernel, on top of which the rest of the OS can be built. For a specific example, the reader may look up the seL4 kernel, a high-performance kernel fully formally verified, a remarkable breakthrough result from the Trustworthy Systems group (TS). A more public show that seL4 was ready for deployment in real-world applications is in the DARPA-funded HACMS program. In that program, seL4 was used to protect an autonomous helicopter against cyber-attacks.
In the more immediate future, there will for sure be many questions about the feasibility, scalability, and adaptability of such a formal approach to AI. Will the dynamic nature of AI, ever-evolving and expanding, fit into this seemingly rigid skeleton of formal language specifications? Can we architect a system where proof assistants don't merely act as gatekeepers, but as enablers of innovation, ensuring AI systems not only comply with regulations but also thrive within their bounds? Many questions, but not many answers at this time.
Could AI Itself Be Used to Formulate and Verify Its Regulations?
With this precision and clarity of democratic governance through formal specifications in mind, we should seriously explore the intriguing possibility of using AI itself, particularly Large Language Models (LLMs), in formulating and verifying these regulations. LLMs, in combination with deductive systems, could assist in mathematical proofing, enhancing their role from mere tools to active participants in their own governance. Notice the twist here, we do not talk only about using math (i.e. proofs) for verifying AI, we talk about AI helping with the math, and the proofs. It’s the dawn of an awesome period.
Towards a Participatory Formal Approach
In the section AI Offers Us a Unique Opportunity to Get Democracy Right of Reader's Guide, we produced a democracy model. The picture below repeats that model and emphasizes the participation arrow.
The formal approach we advocated in this essay refers to the core of the AI systems that would be used in the democracy model above. But perhaps there is no more important aspect of this democracy model that we should be getting right than the participation arrow. How could we design a participatory approach that will be provably not just supportive of but enhancing the most important aspect of the democratic process, the participation of its citizens?
Conclusion
In summary, to harness AI's potential for good, we advocate for a more structured and collaborative (between industry, academia, and government) approach to AI regulation, including stronger formal specifications and proofs of compliance. It is pie in the sky at this time, but we should at least keep that goal alive in our minds.
( Just like all posts have the same diagram at the top, they also have the same set of axioms at the bottom. The diagram at the top is about where we are now, this set of axioms is about the future.
Proposing a formal theory of democratic governance may look dystopian and infringe on a citizen’s freedom of choice. But it is trying to do exactly the opposite, enhancing citizen's independence and avoiding the anarchy that AI intrusion on governance will bring if formal rules for its behavior are not established.
One cannot worry about an existential threat to humanity and not think of developing AI with formal specifications and proving formally (=mathematically) that AI systems do indeed satisfy their specifications.
These formal rules should uphold a subset of democratic principles of liberty, equality, and justice, and reconcile them with the subset of core human values of freedom, dignity, and respect. The existence of such a reconciled subset is postulated in Axiom 2.
Now, the caveat. We are nowhere near such a formal theory, because among other things, we do not yet have a mathematical theory explaining how neural networks learn. Without it, one cannot establish a deductive mechanism needed for proofs. So it will be a long road, but eventually we will have to travel it. )
Towards a Formal Theory of Democratic Governance in the Age of AI
Axiom 1: Humans Have Free Will
Axiom 2: A consistent (=non-contradictory) set of democratic principles and human values exists
Axiom 3: Citizens are endowed with a personal AI assistant (PAIA), whose security, convenience, and privacy are under citizen’s control
Axiom 4: All PAIAs are aligned with the set described in Axiom 2
Axiom 5: A PAIA always asks for confirmation before taking ANY action
Axiom 6: Citizens may decide to let their PAIAs vote for them, after confirmation
Axiom 7: PAIAs monitor and score the match between the citizens’ political inclinations and the way their representatives in Congress vote and campaign
Axiom 8: A PAIA upholds the citizen’s decisions and political stands, and never overrides them
Axiom 9: Humans are primarily driven by a search for relevance
Axiom 10: The 3 components of relevance can be measured by the PAIA. This measurement is private
Axiom 11: Humans configure their PAIAs to advise them on ways to increase the components of their relevance in whatever ways they find desirable
Axiom 12: A PAIA should assume that citizen lives in a kind, fair, and effective democracy and propose ways to keep it as such
More technical justification for the need for formal AI verification can be found on the SD-AI (Stronger Democracy through Artificial Intelligence) website:
articles related to this formalism are stored in SD-AI’s library section